How Machine Learning is Revolutionizing Industries
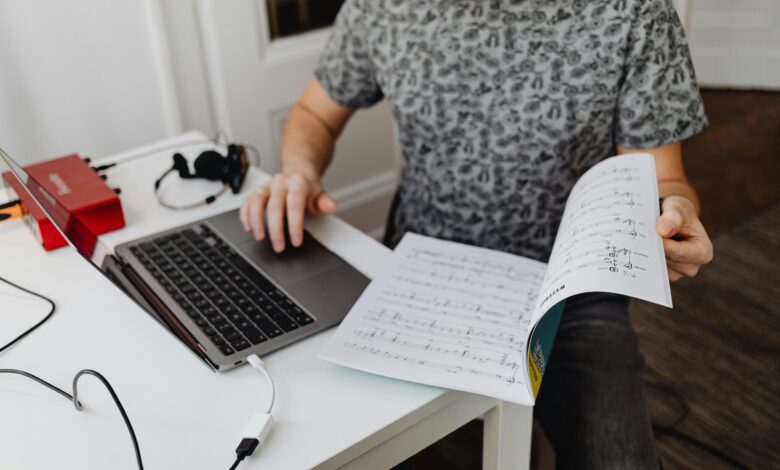
Machine learning (ML), a subset of artificial intelligence (AI), has emerged as one of the most transformative technologies of the 21st century. By enabling systems to learn from data, identify patterns, and make decisions with minimal human intervention, machine learning is reshaping industries across the globe. From healthcare to finance, manufacturing to entertainment, ML is driving innovation, improving efficiency, and creating new opportunities. In this article, we’ll explore how machine learning is revolutionizing various industries and what the future holds.
What is Machine Learning?
Machine learning involves training algorithms to recognize patterns in large datasets and make predictions or decisions based on that data. Unlike traditional programming, where explicit instructions are provided, ML models improve over time as they process more information. Key techniques include supervised learning, unsupervised learning, reinforcement learning, and deep learning.
The widespread adoption of ML is fueled by advancements in computing power, the availability of big data, and improvements in algorithms. These factors have made it possible for businesses and organizations to leverage ML in unprecedented ways.
Industries Transformed by Machine Learning
1. Healthcare
Machine learning is revolutionizing healthcare by enhancing diagnostics, personalizing treatment, and streamlining operations.
- Disease Diagnosis : ML algorithms analyze medical images (e.g., X-rays, MRIs) to detect diseases like cancer, cardiovascular conditions, and neurological disorders with remarkable accuracy.
- Drug Discovery : Pharmaceutical companies use ML to accelerate drug development by predicting molecular interactions and identifying potential compounds faster than traditional methods.
- Personalized Medicine : ML analyzes patient data to tailor treatments based on genetic profiles, lifestyle, and medical history.
- Remote Monitoring : Wearable devices equipped with ML track vital signs and alert healthcare providers to potential issues in real-time.
Example : IBM Watson Health uses ML to assist doctors in diagnosing diseases and recommending treatments.
2. Finance
In the financial sector, machine learning is transforming everything from fraud detection to investment strategies.
- Fraud Detection : ML models analyze transaction patterns to identify anomalies and flag potentially fraudulent activities.
- Algorithmic Trading : Financial institutions use ML to predict market trends and execute trades at optimal times.
- Credit Scoring : ML evaluates creditworthiness by analyzing alternative data sources, such as social media activity or utility payments.
- Customer Service : Chatbots powered by natural language processing (NLP) provide instant responses to customer inquiries.
Example : PayPal uses machine learning to combat money laundering and unauthorized transactions.
3. Retail and E-commerce
Machine learning is redefining the shopping experience by personalizing recommendations, optimizing supply chains, and improving customer engagement.
- Personalized Recommendations : Platforms like Amazon and Netflix use ML to suggest products or content based on user behavior.
- Inventory Management : ML predicts demand, helping retailers optimize stock levels and reduce waste.
- Dynamic Pricing : Algorithms adjust prices in real-time based on factors like demand, competition, and seasonality.
- Visual Search : Tools like Google Lens allow users to search for products using images rather than keywords.
Example : Stitch Fix, an online styling service, uses ML to curate personalized clothing selections for customers.
4. Manufacturing
Machine learning is driving efficiency and innovation in manufacturing through predictive maintenance, quality control, and automation.
- Predictive Maintenance : ML predicts equipment failures before they occur, minimizing downtime and repair costs.
- Quality Assurance : Computer vision systems inspect products for defects during production.
- Supply Chain Optimization : ML forecasts demand, optimizes routes, and manages inventory to ensure timely deliveries.
- Robotics : AI-powered robots perform repetitive tasks with precision, freeing human workers for more complex roles.
Example : General Electric uses ML to monitor jet engines and predict maintenance needs.
5. Transportation and Logistics
The transportation industry is leveraging ML to enhance safety, reduce costs, and improve customer experiences.
- Autonomous Vehicles : Self-driving cars and trucks rely on ML to interpret sensor data and navigate roads safely.
- Route Optimization : Delivery services like UPS use ML to determine the most efficient routes, saving fuel and time.
- Traffic Management : Smart cities employ ML to analyze traffic patterns and optimize signal timing.
- Fleet Management : ML monitors vehicle performance and driver behavior to improve safety and efficiency.
Example : Tesla’s Autopilot system uses machine learning to enable semi-autonomous driving.
6. Marketing and Advertising
Machine learning is transforming marketing by enabling hyper-targeted campaigns and improving ROI.
- Audience Segmentation : ML identifies customer segments based on demographics, behavior, and preferences.
- Ad Targeting : Algorithms serve ads to users most likely to engage, increasing conversion rates.
- Sentiment Analysis : ML analyzes social media posts and reviews to gauge public opinion about brands.
- Content Creation : AI tools generate ad copy, email subject lines, and even video scripts.
Example : Facebook Ads uses ML to deliver highly targeted advertisements to users.
7. Education
Machine learning is personalizing education and making it more accessible.
- Adaptive Learning : Platforms like Duolingo and Khan Academy use ML to adapt lessons to individual students’ needs.
- Automated Grading : ML evaluates essays and assignments, freeing teachers to focus on instruction.
- Dropout Prediction : Schools use ML to identify at-risk students and intervene early.
- Virtual Tutors : AI-powered chatbots provide 24/7 assistance to students.
Example : Carnegie Learning offers adaptive math software that adjusts to each student’s skill level.
8. Agriculture
Machine learning is addressing global food security challenges by optimizing farming practices.
- Crop Monitoring : Drones and satellites equipped with ML analyze crop health and detect pests or diseases.
- Yield Prediction : ML forecasts harvest yields based on weather, soil conditions, and historical data.
- Precision Farming : Sensors and ML guide irrigation, fertilization, and planting schedules.
- Livestock Management : Wearable devices monitor animal health and productivity.
Example : John Deere integrates ML into its tractors to automate planting and harvesting.
9. Entertainment
Machine learning is enhancing content creation, distribution, and consumption in the entertainment industry.
- Content Recommendation : Streaming platforms like Spotify and Hulu use ML to suggest songs, movies, and shows.
- Content Generation : AI creates music, scripts, and visual effects, reducing production costs.
- Audience Insights : ML analyzes viewer behavior to inform creative decisions.
- Deepfake Technology : While controversial, ML enables realistic digital recreations of actors or historical figures.
Example : DeepMind’s WaveNet generates lifelike speech for voice assistants.
Challenges and Ethical Considerations
While machine learning offers immense benefits, it also raises important challenges:
- Bias and Fairness : ML models can perpetuate biases present in training data, leading to unfair outcomes.
- Privacy Concerns : The collection and analysis of vast amounts of personal data raise privacy issues.
- Job Displacement : Automation may lead to job losses in certain sectors, necessitating workforce reskilling.
- Transparency : Many ML algorithms operate as “black boxes,” making it difficult to understand their decision-making processes.
Addressing these concerns requires collaboration between governments, businesses, and researchers to establish ethical guidelines and regulatory frameworks.
The Future of Machine Learning
As machine learning continues to evolve, its impact will only deepen. Emerging trends include:
- Explainable AI : Efforts to make ML models more transparent and interpretable.
- Edge Computing : Running ML algorithms on local devices to reduce latency and enhance privacy.
- Quantum Machine Learning : Leveraging quantum computing to solve complex problems faster.
- Human-AI Collaboration : Integrating ML into workflows to augment human capabilities rather than replace them.
By embracing these advancements responsibly, industries can unlock the full potential of machine learning while mitigating risks.